A Computer Spotted a Turtle Hiding Out in a Cloud of 'Quantum Fireworks'
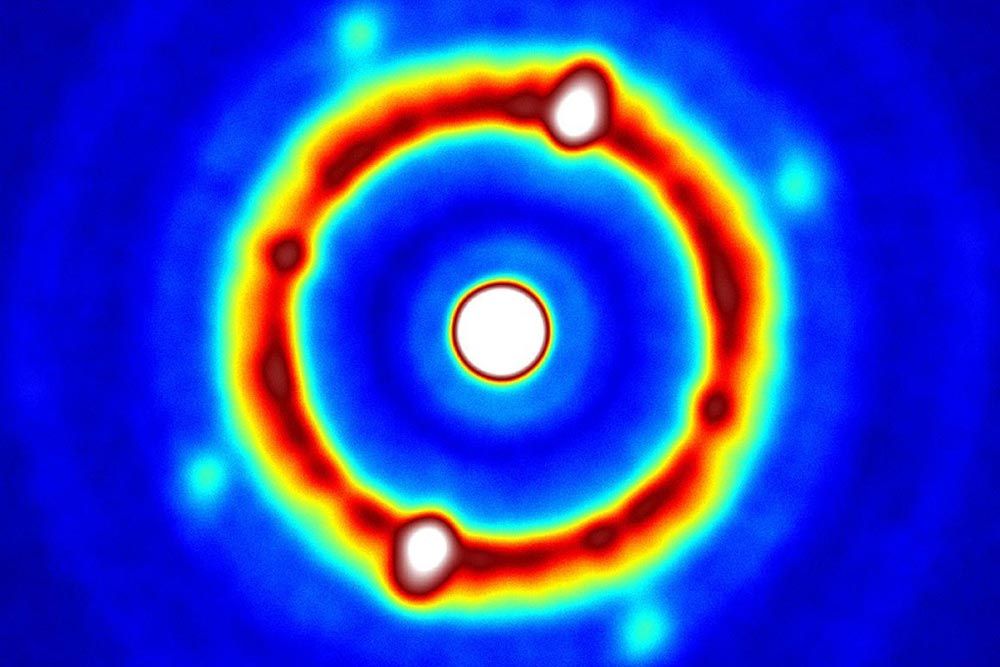
Zap a mass of supercooled atoms with a magnetic field and you'll see "quantum fireworks" — jets of atoms firing off in apparently random directions.
Researchers discovered this back in 2017, and they suspected there might be a pattern in those fireworks. But they couldn't spot it on their own. So, they turned over the problem to a computer trained in pattern matching, which was able to spot what they couldn't: a shape, painted by the fireworks over time, in blast after atomic jet blast. That shape? A funky little turtle.
The results, published as a report Feb. 1 in the journal Science, are among the first major examples of scientists using machine learning to solve quantum-physics problems. Folks should expect to see more digital assists of this sort, the researchers wrote, as quantum-physics experiments increasingly involve systems too large and complex to analyze using brainpower alone. [The 18 Biggest Unsolved Mysteries in Physics]
Here's why the computerized help was necessary:
To create the fireworks, the researchers started with a state of matter called a Bose-Einstein condensate. That's a group of atoms brought to temperatures so close to absolute zero that they clump together and start to behave like one superatom, exhibiting quantum effects at relatively large scales.
Each time a magnetic field struck the condensate, a handful of atomic jets would shoot away from it, in apparently random directions. The researchers made images of the jets, pinpointing the atoms' positions in space. But even lots of those images layered on top of each other didn't reveal any obvious rhyme or reason to the atoms' behavior.
What the computer saw that humans couldn't was that if those images were rotated to sit on top of one another, a clear picture did emerge. The atoms on average tended to fling themselves away from the fireworks in one of six directions relative to one another during each blast. The result was that enough images, rotated and layered in the right way, revealed four "legs" at right angles to each other, as well as a longer "head" between two of the legs matched with a "tail" between the other two. The rest of the atoms were pretty evenly distributed across three rings, which made up the turtle's shell.
Get the Space.com Newsletter
Breaking space news, the latest updates on rocket launches, skywatching events and more!
This wasn't obvious to human observers because the direction in which the "turtle" was oriented during each blast was random. And each blast made up only a few pieces of the overall turtle-shaped puzzle. It took a computer's infinite patience for sifting through messy data to figure out how to arrange all the images such that the turtle emerged.
This sort of method — turning a computer's pattern-recognition abilities loose on a big, messy data set — has been effective in efforts ranging from interpreting the thoughts passing through human brains to spotting exoplanets orbiting distant stars. It doesn't mean computers are outpacing humans; people still have to train the machines to notice the patterns, and the computers don't in any meaningful way understand what they're seeing. But the approach is an increasingly widespread tool in the scientific tool kit that has now been applied to quantum physics.
Of course, once the computer turned up this result, the researchers checked its work, using some old-fashioned pattern-hunting techniques already common in quantum physics. And once they knew what to look for, the researchers found the turtle again, even without the computer's help.
None of this research yet explains why the fireworks, over time, exhibit the turtle shape, the researchers pointed out. And that's not the sort of question machine learning is well-suited to answer.
"Recognizing a pattern is always the first step in science, so this type of machine learning could identify hidden relationships and features, especially as we shift to try to understand systems with a large number of particles," lead author Cheng Chin, a physicist at the University of Chicago, said in a statement.
The next step in figuring out why those fireworks make a turtle pattern will likely involve a lot less machine learning and a lot more human intuition.
Originally published on Live Science.
Join our Space Forums to keep talking space on the latest missions, night sky and more! And if you have a news tip, correction or comment, let us know at: community@space.com.
Rafi wrote for Live Science from 2017 until 2021, when he became a technical writer for IBM Quantum. He has a bachelor's degree in journalism from Northwestern University’s Medill School of journalism. You can find his past science reporting at Inverse, Business Insider and Popular Science, and his past photojournalism on the Flash90 wire service and in the pages of The Courier Post of southern New Jersey.